This course introduces the theoretical, philosophical, and mathematical foundations of Bayesian Statistical inference. Students will learn to apply this foundational knowledge to real-world data science problems. Topics include the use and interpretations of probability theory in Bayesian inference; Bayes’ theorem for statistical parameters; conjugate, improper, and objective priors distributions; data science applications of Bayesian inference; and ethical implications of Bayesian statistics.

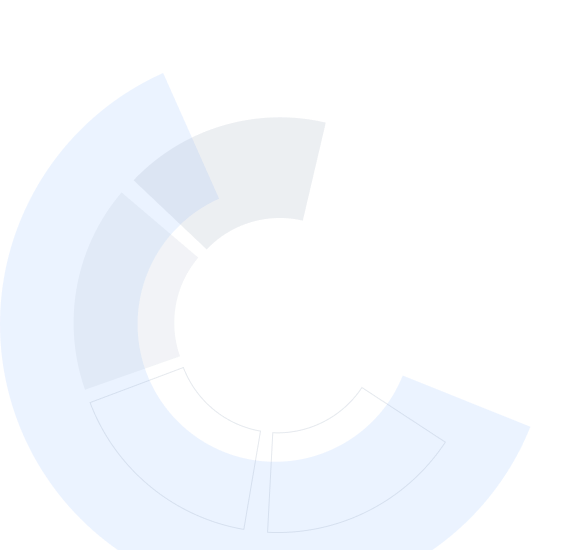
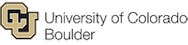
Recommended experience
What you'll learn
Implement Bayesian inference to solve real-world statistics and data science problems.
Articulate the logic of Bayesian inference and compare and contrast it with frequentist inference.
Utilize conjugate, improper, and objective priors to find posterior distributions.
Details to know

Add to your LinkedIn profile
May 2025
8 assignments
See how employees at top companies are mastering in-demand skills
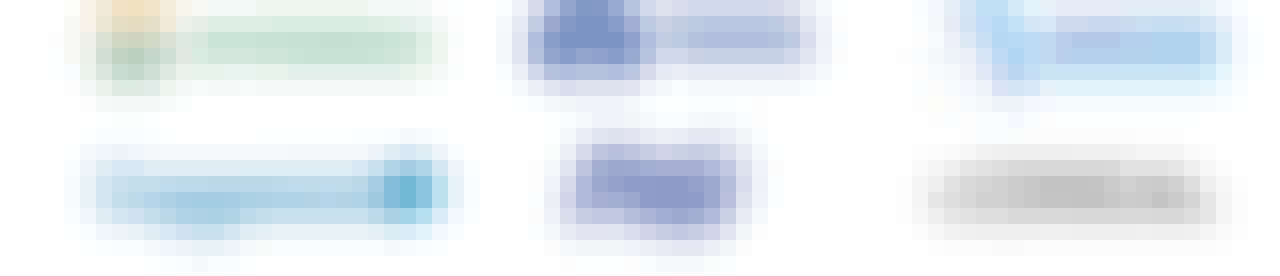
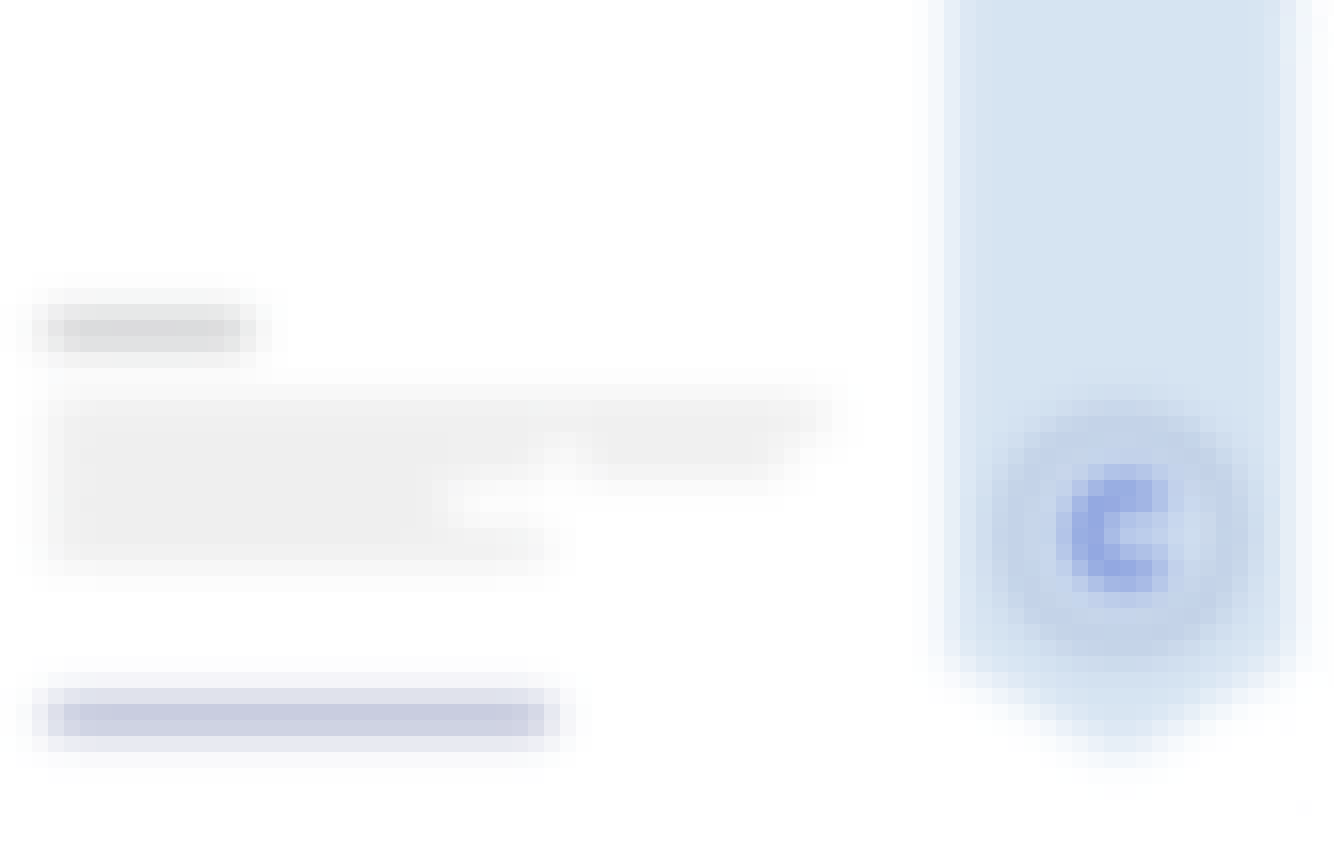
Earn a career certificate
Add this credential to your LinkedIn profile, resume, or CV
Share it on social media and in your performance review
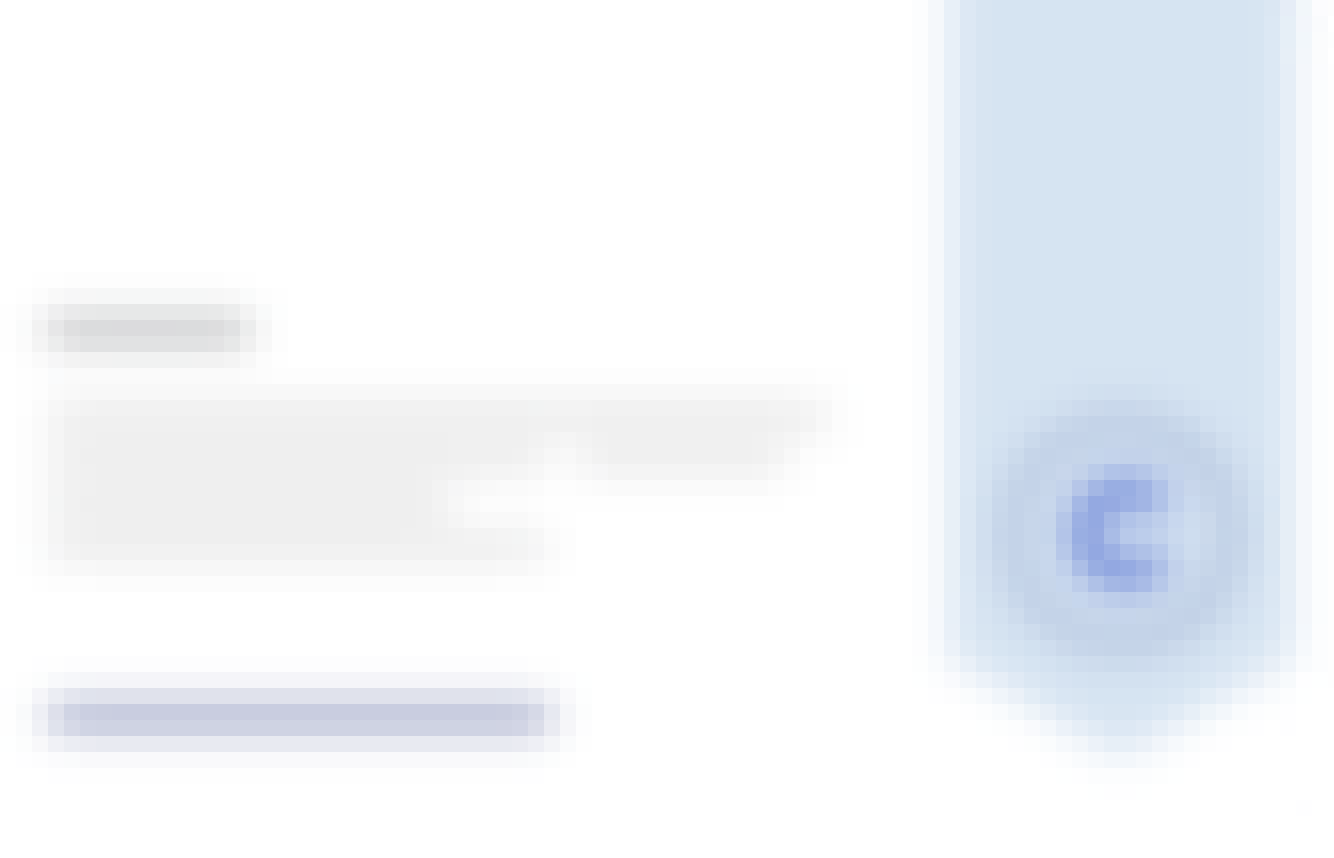
There are 6 modules in this course
This module introduces learners to Bayesian statistics by comparing Bayesian and frequentist methods. The introduction is motivated by an example that illustrates how different assumptions about data collection - specifically, stopping rules - can result in different conclusions when using frequentist methods. Bayesian methods, on the other hand, yield the same conclusion regardless of stopping rules. This example illuminates a key philosophical difference between frequentist and Bayesian methods.
What's included
8 videos7 readings2 assignments3 programming assignments1 discussion prompt2 ungraded labs
This module introduces learners to Bayesian inference through an example using discrete data. The example demonstrates how the posterior distribution is calculated and how uncertainty is quantified in Bayesian statistics. The module also describes methods for summarizing the posterior distribution and introduces learners to the posterior predictive distribution through use of the Monte Carlo simulation. Monte Carlo simulations will be important for advanced computational Bayesian methods.
What's included
6 videos1 assignment1 programming assignment2 ungraded labs
This module introduces learners to methods for conducting Bayesian inference when the likelihood and prior distributions come from a convenient family of distributions, called conjugate families. Conjugate families are a class of prior distributions for which the posterior distribution is in the same class. The module covers the beta-binomial, normal-normal and inverse gamma-normal conjugate families and includes examples of their application to find posterior distributions in R.
What's included
7 videos1 reading1 assignment1 programming assignment2 ungraded labs
This module motivates, defines, and utilizes improper and so-called "objective" prior distributions in Bayesian statistical inference.
What's included
7 videos1 reading1 assignment1 programming assignment2 ungraded labs
In this module, learners will be introduced to Bayesian inference involving more than one unknown parameter. Multiparameter problems are motivated with a simple example: a conjugate prior, two-parameter model involving normally distributed data. From there, we learn to solve more complex problems, including Bayesian linear regression and variance-covariance matrix estimation.
What's included
9 videos1 reading1 assignment1 programming assignment3 ungraded labs
This module contains materials for the proctored final exam for MS-DS degree students. If you've upgraded to the for-credit version of this course, please make sure you review the additional for-credit materials in the Introductory module and anywhere else they may be found.
What's included
6 readings2 assignments
Instructor

Offered by
Why people choose Coursera for their career




New to Probability and Statistics? Start here.

Open new doors with Coursera Plus
Unlimited access to 10,000+ world-class courses, hands-on projects, and job-ready certificate programs - all included in your subscription
Advance your career with an online degree
Earn a degree from world-class universities - 100% online
Join over 3,400 global companies that choose Coursera for Business
Upskill your employees to excel in the digital economy
Frequently asked questions
Access to lectures and assignments depends on your type of enrollment. If you take a course in audit mode, you will be able to see most course materials for free. To access graded assignments and to earn a Certificate, you will need to purchase the Certificate experience, during or after your audit. If you don't see the audit option:
The course may not offer an audit option. You can try a Free Trial instead, or apply for Financial Aid.
The course may offer 'Full Course, No Certificate' instead. This option lets you see all course materials, submit required assessments, and get a final grade. This also means that you will not be able to purchase a Certificate experience.
When you enroll in the course, you get access to all of the courses in the Specialization, and you earn a certificate when you complete the work. Your electronic Certificate will be added to your Accomplishments page - from there, you can print your Certificate or add it to your LinkedIn profile. If you only want to read and view the course content, you can audit the course for free.
If you subscribed, you get a 7-day free trial during which you can cancel at no penalty. After that, we don’t give refunds, but you can cancel your subscription at any time. See our full refund policy.
More questions
Financial aid available,